Analyzing Betting Outcomes With Monte Carlo Simulation
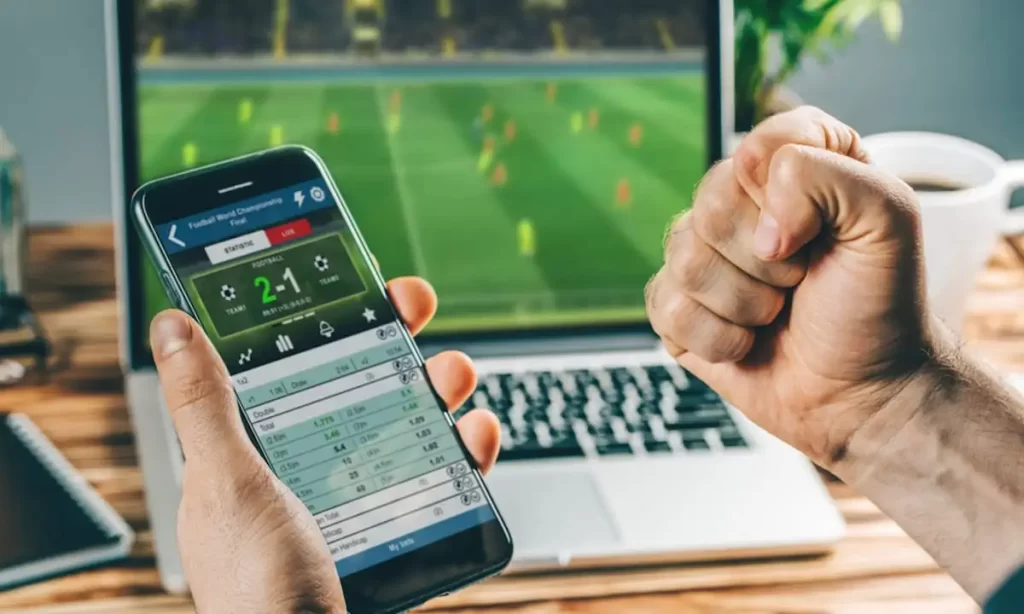
MCS is an efficient decision-making method when describing complex and volatile systems. It can be used in finance as well as project management.
Random sampling and dozens of iterations necessary to make correct prediction. By creating multiple outcomes at random, this approach creates an in-depth picture of risk, providing an in-depth picture of it as well as providing better forecasting and more precise predictions.
Variables
Monte Carlo simulations can assist professionals from different fields assessing risk in various forms, whether that’s a banker evaluating potential toxic chemicals in South Korean cabbage kimchi or telecom service provider assessing its ability to handle viewer traffic during the Olympics. The techniques, named for Monaco’s gambling capital, are increasingly utilized in financial planning and decision-making practices.
Monte Carlo simulations use Monte Carlo sampling to approximate actual probability distributions more closely, making them useful in analyzing project outcomes and communicating them to stakeholders. They also help teams prioritize critical tasks or high-risk areas of a project while creating contingency plans – all strategies which improve overall project performance while decreasing uncertainty.
Simulations
Monte Carlo simulations offer an alternative way of gathering data by simulating inputs and their effects on outputs; then this data is statistically analyzed to provide predictions or estimates for any particular problem.
Beginning by creating a model that represents business activity, plan or project; next identifying random variables with their distributions; the model then executes random numbers many times to simulate potential outcomes before gathering and analyzing using statistical software such as Excel or Lumivero’s @RISK to produce predictions, standard deviations and variances as well as charts and graphs.
Business professionals across industries rely on Monte Carlo simulation to accurately assess risks and make confident decisions with ease. Common uses for Monte Carlo simulation include performance testing (such as how a web app handles increased traffic or responds to scaling), as well as tracking possible price movements of assets through pricing stock options.
Outcomes
One key to successfully employing a Monte Carlo simulation is gathering accurate data. This includes team statistics, player performance measurements and external factors like weather and venue. High-quality information helps determine probabilities associated with different outcomes.
Monte Carlo simulations simulate possible outcomes of any given scenario by employing probability distributions for input factors and model parameters, then randomly sampling from these distributions thousands of times to arrive at their average. This provides analysts with an accurate view of potential outcomes and their likelihood. Developed as part of World War II as part of the Manhattan Project by mathematician Stanislaw Ulam and computer scientist John von Neumann as the Monte Carlo method was named for Monaco due to its emphasis on chance and randomness.
Outliers
The Monte Carlo model typically yields better results than a traditional betting model, but the variable cannot be analyzed to any 100% accuracy (weather or any other external factor). For them to be attenuated, for the adverse impact these variables might have, as much good data as we can find should be compiled – team statistics, player statistics, last matches and any other details of importance.
Monte Carlo simulations are most often employed in the financial industry; however, they can also be applied to virtually every other profession that involves measuring risk and planning accordingly. They’re particularly helpful for estimating the probability of gain or loss when investing. Plus, advanced analysis techniques like optimization algorithms and sensitivity analyses can further increase accuracy when applied alongside Monte Carlo simulations.
Analysis
Monte Carlo simulation provides you with an effective tool for making decisions under uncertainty by helping you understand probabilities and identify value. That’s basically building a model of an event, randomizing input variables to within the parameters that are likely to fit – that then gives you hundreds or thousands of possible results and you try to find the probabilities for those results.
An analysis can also help you determine your risk tolerance, which is essential when placing bets. With its results you can find strategies with different risk levels to maximize profit potential and identify strategies with variable risks levels that work well for you. In addition to betting, this technique has many applications besides simply betting, including project management, cost estimation and stock option pricing. Combined with artificial intelligence (AI), its accuracy and speed can also be enhanced – an indispensable asset for professionals dealing with uncertainty.